Transfer Learning with Self-Supervised Vision Transformers for Snake Identification
Sep 12, 2024·
,,·
0 min read
Anthony Miyaguchi

Murilo Gustineli
Austin Fischer
Ryan Lundqvist
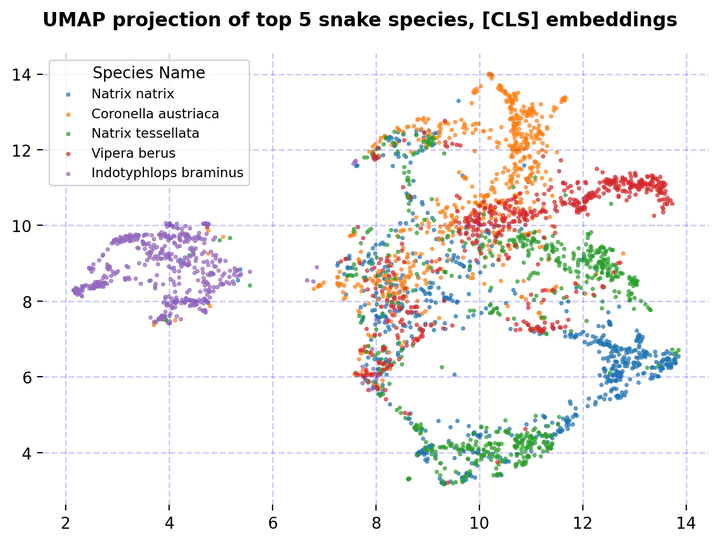
Abstract
We present our approach for the SnakeCLEF 2024 competition to predict snake species from images. We explore and use Meta’s DINOv2 vision transformer model for feature extraction to tackle species’ high variability and visual similarity in a dataset of 182,261 images. We perform exploratory analysis on embeddings to understand their structure, and train a linear classifier on the embeddings to predict species. Despite achieving a score of 39.69, our results show promise for DINOv2 embeddings in snake identification. All code for this project is available at https://github.com/dsgt-kaggle-clef/snakeclef-2024.
Type
Publication
CEUR Workshop Proceedings (CEUR-WS.org)